Utilizing a quantum annealing approach and different encoding methods within lattice protein folding for drug discovery

Just carrying on with normal life. You are happy, have a nice family and job. When going on with your regular activities one day, you begin to feel short of breath, when suddenly you feel a freezing numbness on the left side of your face and leg. You begin to lose control of your senses while this obscure mask is slowly taking over, and the next time you wake up, you’re in a hospital learning that you experienced your first stroke.
The first only marks the beginning however, as more strokes follow, and eventually when the doctors figure out the source of all this, you are diagnosed with cardiovascular disease, and you have less than two years to live.
Everything you’ve worked hard for this moment, all your future ambitions and goals, just suddenly snatched away and gone.
The truth is, many people around the world experience this, and I was able to second-handedly experience this from a few close members of my family. In the US alone, over 640,000 people die of heart disease each year. That’s equivalent to one person dying of heart disease every 40 seconds.
And it’s incredibly surprising that despite the tremendous number of people who are affected by this, as well as the time we’ve spent researching, we still haven’t found a cure.
What now?
But what if drug discovery and the designing of new protein-based drugs were able to happen more efficiently and at a much faster rate? Not only will this help the millions of people diagnosed with these diseases and illnesses, but will also assure their families and close ones.
This approach utilizes quantum-based algorithms to fold long protein sequences, where lattice models are then used to examine the specific properties of protein folding and design.
Today, the conventional way of testing out these sequences are with the “take and test” method, by trying out each candidate until you generate a list of possible solutions, or in other words, “maybe candidates.” However, this method is extremely inefficient, where finding the “right candidate” can take a matter of years, accounting for a reason why drug development is so slow and tedious. Classical machine learning methods are also carried out, but are extremely restricted to due to computational size limits.
This is where quantum comes in, providing an extremely efficient and accurate mapping of these proteins. By using optimization techniques, the quantum method is able to find the most suitable candidate within a matter of weeks. These algorithms also have a high potential in mapping out the complex relationships between these proteins, providing a huge advantage over its classical counterpart (who doesn’t have enough computing power to operate through simulating the folds).
By mapping out the relationships, we develop a better understanding of these protein functions, which can lead to new drug designs for tackling various diseases.
Proteins and the Protein Folding Problem
Proteins, as a basis, are the building blocks of everything in the universe. From repairing our body’s tissues to coordinating our bodily functions and maintaining pH balance, they provide a strong structural framework essential to our survival.
A protein molecule is formed by a string of small components, known as amino acids, which are eventually able to fold into the molecule’s native 3D shape. The 20 different types of amino acids and their combinations add up to a particular fold to create these proteins, the shape they eventually turn into directly correlating to its function.

In the case that a protein does not fold into their native (or correct) state, the “failed” one could lead to a misfolded protein. This in turn could lead to extremely toxic effects, including neurodegenerative diseases and a weakened immune system. When addressing these misfolded proteins, we are still unsure of getting to the formation through folding even though we are familiar with the protein structure itself,.
This challenge of figuring out how a string of amino acids can encode the 3D shape of a protein, is better known as the protein folding problem.
The Protein Folding Problem

One of the grandest problems in molecular biology and biochemistry today is understanding the folding of macromolecules, specifically proteins. With proteins, what you are given is this one giant 20-letter word where you are then assigned a task to predict the global, three-dimensional structure that these proteins fold into.
From a chain of letters to a 3D structure? Let’s just say this process is extremely difficult. So with the 20 available amino acids, each having varying molecular properties, when expanding to the human protein ranging to around 400 amino acids long, the computational complexity is huge.
The hunt for finding an efficient way into folding proteins into their desired shapes has been overwhelming scientists for the past 50 years.The real puzzle here is finding the folding pattern of a misfolded protein. We already have success into simulating a protein folding into their native state, but if we’re able to model these unfolded proteins, this enables us to find the cure to a variety of diseases, including Alzheimer’s, Parkinson’s and Huntington’s.
Since the shape of a protein dictates its function in the body, by being able to predict a protein’s structures, this allows scientists to form new protein-based drugs to treat diseases.
If we spent over 50 years researching this, we had to have made at least some progress, right? The answer is yes, we have, but we still have a long way to go until we are able to fully understand all these proteins and the complex relationships that exist with them.
Lattice Protein Folding
Modeling techniques for protein folding today include lattice protein folding, which as a result, provide an extremely simplified version of the different amino acid combinations.
Lattices are any grid of regularly spaced points stretching to infinity. The points are also known as vertices which connect to other vertices in the lattice by the edges.

The lattice protein fold is defined as a path in the graph of a given grid, which passes through a vertex only once, while assigning an amino acid type to each vertex passed through.
With lattice protein folding, the simplification is a twofold process where each whole amino acid (also known as residue) represents a single bead of a defined set of types. Each residue is restricted as it is placed on the vertices of a cubic lattice. This in turn, aims to minimize the free energy of the contacts between hydrophobic amino acids, which are predicted to group around each other.

Lattice proteins introduce an energy function, which is a set of conditions specifying the interaction energy between the points that occupy different lattice sites. This in turn is made to resemble real proteins. The energy function then mimics the steric or hydrogen bonding effects within the amino acids of the proteins. The bead is then split into types, with the energy function specifying the reaction depending on the bead type.
For example, the hydrophobic-polar model features two beads: hydrophobic and polar, while mimicking the hydrophobic effect by specifying an interaction between the H beads. The energy of the protein fold is calculated as the sum of interaction energies between adjacent amino acids.
Conventional Approach to Protein Folding
Utilizing the trial-and-error with classical machine learning
The conventional way in determining the shapes of these proteins are by using experimental techniques, including cyco-electron microscopy and X-ray crystallography, but many drawbacks and costs are included in this trial-and- error method.

A popular newly-developed approach today is using machine learning to predict protein structures and protein fold recognition from amino acid sequences. The core of this is a neural network that is fed with data on how amino-acid sequences are able to map out protein structures. It then learns to produce new structures from unfamiliar sequences by predicting the structure of a protein segment, modeling based on what came before and after it. These deep neural networks then predict the distances between different pairs of amino acids and the angle between their chemical bonds.
This new method is only effective to a certain extent. Time and efficiency are two major obstacles to address, with computational power as an additional difficulty. With the Levinthal Paradox, it takes more than the age of the universe (14 billion years) to try all the possible combinations of protein folding based on amino acid chains, while the body only takes seconds to achieve the folding pattern. At this rate, we can never effectively map out these proteins!

What if these proteins increase in size? This is another disadvantage, as the bigger the protein, the more interactions between the amino acids and the more difficult to model. Proteins larger than 150 residues are unable to be computed classically, and it is still not possible to fold all real protein combinations into a computer.
Currently, no classical algorithm exists that is able to find the lowest energy state of a lattice protein within polynomial time, further restricting the use of lattice folding for smaller protein lengths.
Protein folding continues to be a problem, but can potentially be solved through quantum computing, and more specifically through quantum annealing. The lowest energy path when creating a protein can be found much more efficiently, without being limited to the amount of classical computational power.
Quantum Computing Approach
The quantum annealing method and ways of encoding the qubits for protein folding and modeling
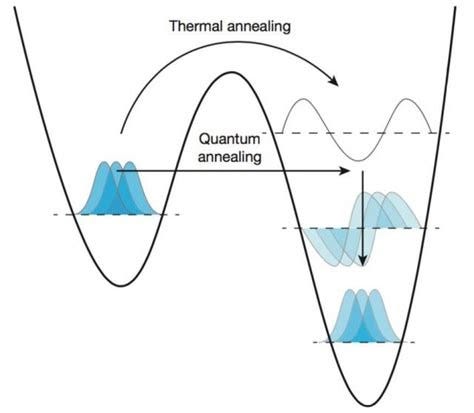
This specific quantum approach uses annealing for leveraging Ising-type Hamiltonians in order to fold protein sequences along a lattice model. By preparing a sub-group of qubits into their lowest-possible energy, it performs a series of operations to be put into a more complex ground state, which cannot easily be solved with classical methods.
The final model will consist of mathematical representations of amino acids within a lattice that are connected by different interaction strengths. By utilizing the process of annealing, the lowest configurations of amino acids and interactions are found, corresponding to the folding of the lattice proteins, in sizes simply unreachable with classical hardware.
The steps to solving the lattice protein folding problem by utilizing quantum annealing is through:
- Constructing the injective mapping between the set of all possible lattice protein folds and binary strings (represented by the qubits). This fully decodes a solution string into the lattice protein fold.
- Constructing the energy landscape for the model so that the lowest energy conformation of the lattice protein corresponds to the system’s ground state. This is done by using pseudo-boolean expressions which prevents overlapping or disconnected amino sequences.
By mapping out these lattice proteins to binary, a binary coordinate system is imposed to a cubic 3D lattice. To make this process even more efficient (in terms of resources, by decreasing the number of qubits used), turns, or defined directions that encode the proteins onto the lattice graph is included. By fixing the first three qubits and giving a ‘right’ move, we are able to fix two more qubits in the second turn, giving a solution bit string.
The binary encoding method would later be used for turn ancilla and turn circuit encoding.
Turn Ancilla Encoding
Turn Ancilla Encoding puts ancillary qubits into the Hamiltonian in order to encode information about the amino acid reactions. The turn ancilla mapping requires this number of qubits for a length N protein:

The Hamiltonian that is then constructed with the turn ancilla scheme consists of four subcomponents: H-back, H-redun, H-olap, and H-pair.

- The H-back component: marks the invalid proteins to ensure the ground state does not have these properties. If two consecutive edges within a protein fold go in between the same pair of vertices, it penalizes the lattice protein fold.
For example, if an edge is represented by vertices (v2, v3) and is followed by another edge (v3, v1), this is invalid as protein “goes back on itself.”
- The H-redun component: marks and penalizes when two 3-bit strings are of the same value, ast hey don’t encode any valid direction within the grid.
- The H-olap component: Similar to the H-back component, but its function is to reduce the number of required ancillary qubits.
- The H-pair component: marks interaction between non-bonded acids adjacent on a lattice.
Turn circuit encoding
Turn circuit encoding is considered the most efficient qubit mapping, requiring only a few number of qubits (compared to other ways) to encode a protein length N.
The turn circuit encoded Hamiltonian is displayed, consisting of the two main terms: H-olap and H-pair.

They are also known as sum strings of each pair of amino acids, able to test for any possible overlaps or interactions of residues. These sum strings are computed by using half-adder circuits, taking two binary numbers x and y as inputs, and outputting the two-bit sum of x and y. In this scenario, the half-adder circuit results in a sum string containing the number of turns the protein takes in the (k) direction between any two residues.

Binary Flag based encoding
An alternative method to encoding the coordinates of the vertices of proteins is by using labels, each represented with every possible position of an amino acid. A linear mapping for the number of qubits with the size of the lattice space being bounded by the radius.
Nested Shell Encoding
The nested shell encoding method is based on two ideas 1) enforcing an arbitrary bound on the radius of the lattice space, allowing the lattice fold to “explore” and 2) using qubits as binary flags as markers for the location of an amino acid instead of directly encoding the information about their position.
The Hamiltonian for the nested shell encoding is given:

In this case, H-one makes sure that no two qubits for the same amino acid are signaling at the same time, and H-olap, providing the constraints to prevent two qubits from different amino acids in claiming the same vertex.
The use of lattice models allow us to investigate these relationships of protein structure and sequences. Different methods of encoding, including ancilla, turn circuit, binary-flagged based, and nested shell are able to support multi-atom representations of these amino acids and many-body energy functions. This in turn allows a higher representation accuracy for protein structure (in the secondary and tertiary levels) as well as enabling faster calculations.
Implications and Drug Discovery

These annealing methods within quantum computing are still relatively new, and based on the great progress we’ve made in the past few years, we will soon be able to improve our understanding of the human body, where we can then target and design new cures for diseases much more efficiently.
Currently, scientists mapped the structures for around half of the proteins made in human cells. With the quantum annealing method, as it continues to develop, will help with the prediction of the protein folding and shapes, much more rapidly and economically, compared to conventional trial and error methods or classical machine learning tools.
As we’re able to instantly gain more knowledge about these proteins shapes and how they operate through these simulations and models, it can then lead to efficient drug discovery. The designing of these protein-based therapeutic drugs, ranging from treatments in anemia, pulmonary treatment, strokes, breast and lung cancers will save these tens of millions of lives from these related deaths.
Not only will protein folding contribute to drug discovery, but its implications even range into advances in biodegradable enzymes by protein design. As we’re on our way to a more eco-friendly environment, it could help manage pollutant (plastic and oils) while also breaking down waste easier, being more beneficial to the environment.
With continual progress being made in this field of quantum computing, its potential ranges from two of the biggest challenges we face today, with drug discovery to finding more environmentally-friendly solutions. This only marks the beginning, we can only imagine what the future implications are for this field in the upcoming years.
If you liked this article, add a clap and stay in tuned for more articles coming soon! Reach out to me at aliceliu2004@gmail.com or on LinkedIn